Semantic Communications |
In the 2030s, the demand for higher data rate will increase to accommodate advanced services such as virtual reality and fully autonomous driving. In order to solve this problem, in the past, a wider bandwidth such as mmWave and THz was utilized. However, semantic communication is not about simply increasing amount of resource, but about how to use limited resources intelligently and efficiently.
|
While the existing Shannon communication paradigm focused on accurately receiving each symbol or bit, semantic communication only needs to deliver intent information of the source to the receiver. Then irrelevant data can be ignored, which can be the key to saving bandwidth, latency and energy by significantly reducing the amount of data to be transmitted or recovered. Thus, in contrast to the Shannon paradigm, semantic communication can lead to next-generation communication systems that greatly reduce data traffic because the receiver transmits only the necessary information related to a specific task.
|
|
|
Research Topics and Broader Impact |
(1) Semantic communication for image transmission |
(2) Semantic communication for text transmission |
(3) Semantic communication for speech transmission |
(4) Broader Impact |
|
|
|
(1) Semantic communication for image transmission |
Semantic communications only transmit necessary information relevant to the specific task at the receiver, which leads to a truly intelligent system with significant reduction in data traffic. The figure below demonstrates the concept of semantic communications, where the transmission task is image recognition. Instead of transmitting bit sequences representing the whole image, a semantic transmitter extracts features relevant to recognize the object, i.e., dog, in the source. The irrelevant information, such as image background, will be omitted to minimize the transmitted data without degrading performance. As a result, the demands on energy and wireless resources will be lowered significantly, leading to a more sustainable communication network. |
|
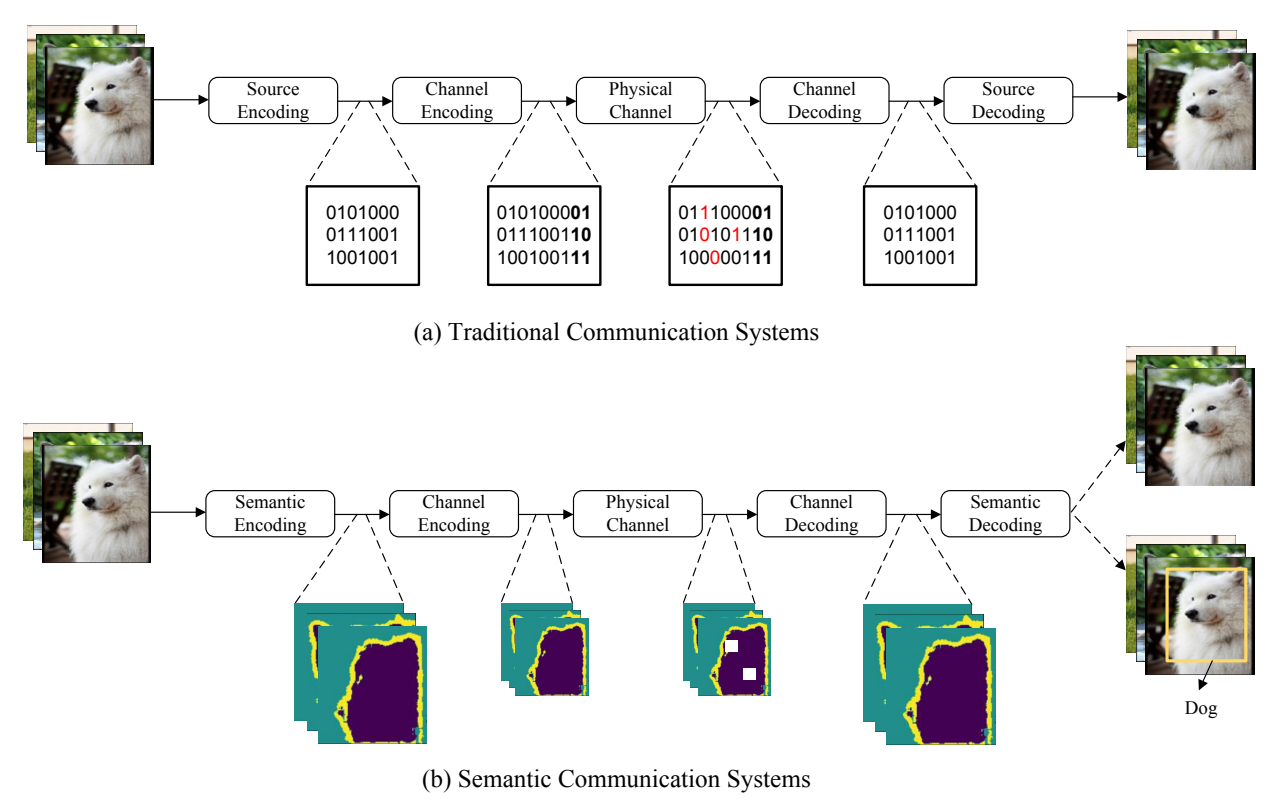 |
|
|
|
(2) Semantic communication for text transmission |
For the text transmission in semantic communication as shown in the figure below, the agents involved in transmitting and receiving messages are expected to do more than just act as basic communication terminals. These agents can be humans, machines, or other intelligent devices and are able to perceive their environment and operate independently. The source agent is responsible for extracting the meaning from raw messages and encoding it into symbols for transmission. The target agent must be capable of understanding and inferring the intended meaning of these messages, even when dealing with subtle differences in meaning. For instance, natural language systems use syntax to help the agents understand the meanings of words in sentences, and agents must be able to recognize synonyms and distinguish between different meanings of words despite differences in syntax, synonyms have the same meaning in a semantic sense. |
The latest advancements in natural language processing (NLP) and deep learning (DL) have made it possible to implement semantic encoding/decoding and channel encoding/decoding using deep neural networks (DNNs). In this system, an auto-encoder and auto-decoder serve as the semantic source and destination, performing both semantic encoding/decoding and channel encoding/decoding together. Compared to traditional communication systems, this approach can achieve a global optimality.
|
|
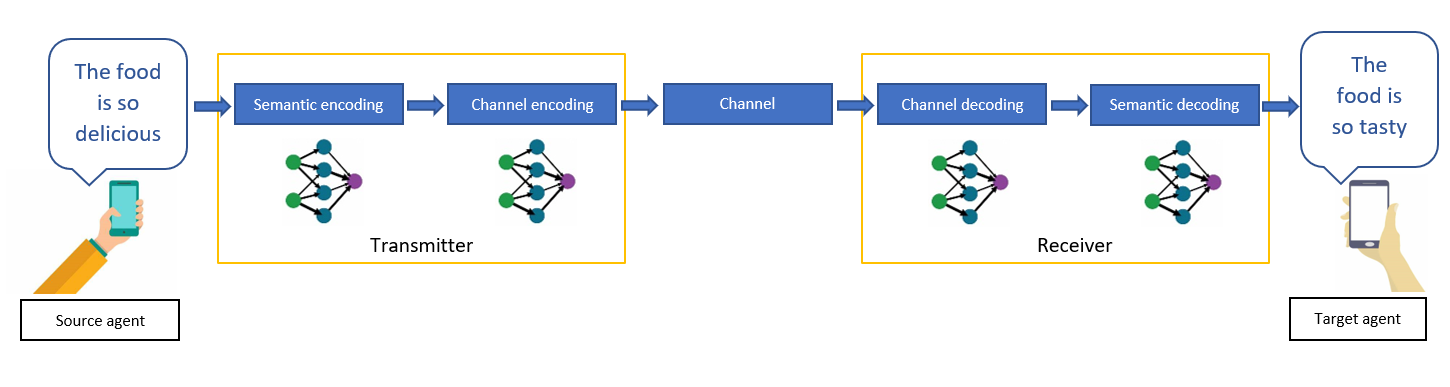 |
|
|
|
(3) Semantic communication for speech transmission |
Semantic communication for speech transmission refers to the process of conveying meaning and understanding between a speaker and a listener through spoken language. It involves the use of words, phrases, and other linguistic symbols to express ideas, thoughts, and emotions, and relies on shared knowledge and understanding between the speaker and the listener. One of the key challenges in this is the potential for misinterpretation or misunderstanding due to ambiguity in language or cultural differences between the speaker and the listener. Another common problem is the degradation of speech signals due to noise, reverberation, or other environmental factors, which can make it difficult to extract meaningful information from the audio signal. To address these issues, advances in machine learning and artificial intelligence have the potential to revolutionize semantic communication by enabling more accurate and efficient processing of natural language, as well as facilitating real-time translation and interpretation between different languages and cultures. |
|
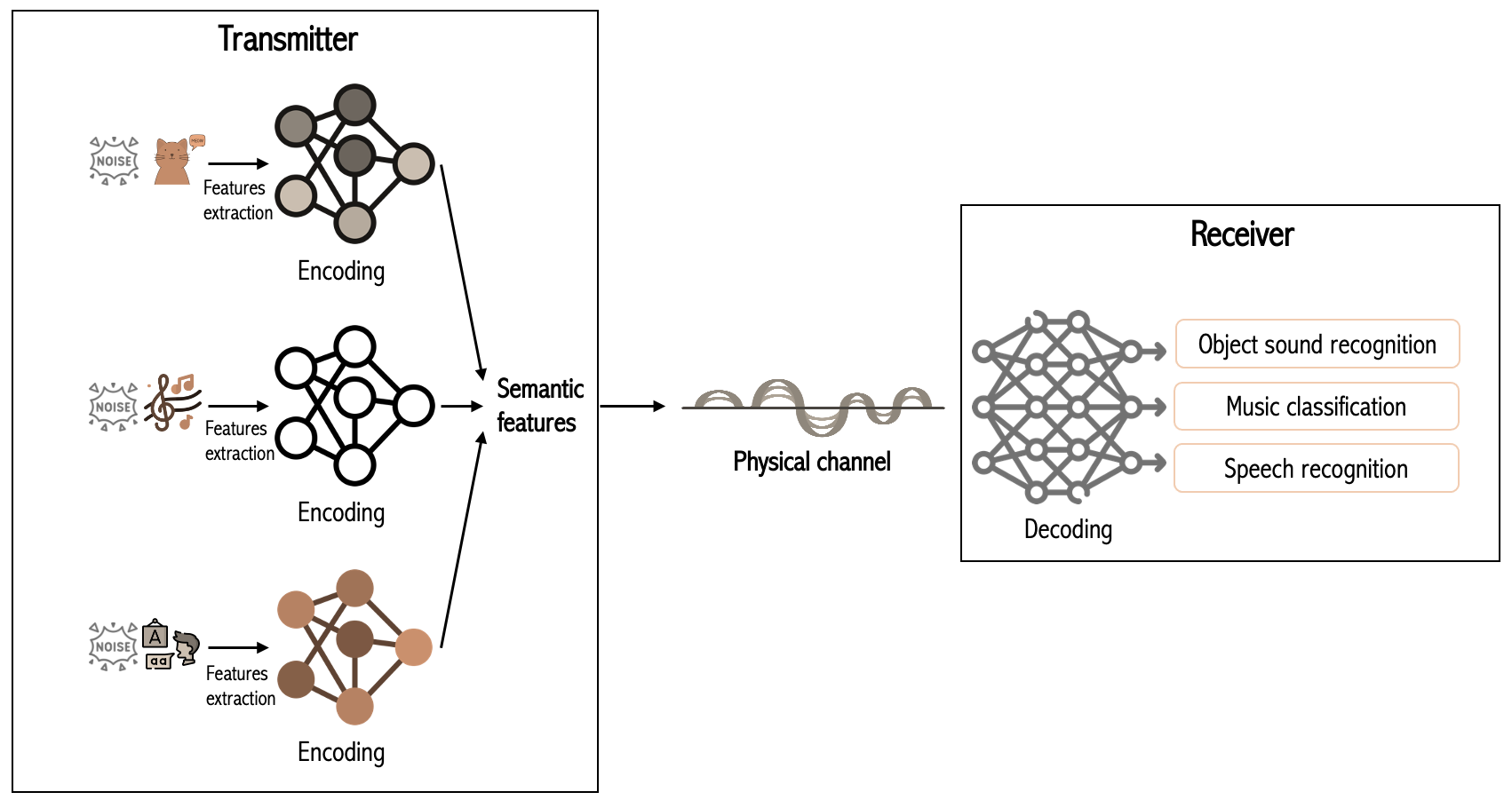 |
|
(4) Broader Imapct |
The project is expected to pave the way for the development and research of semantic communication. The resulting technologies greatly reduce the data traffic by intelligently and efficiently using limited network resources. The research is able to exploit and demonstrate the efficiency and effectiveness of technologies about semantic communication. |
|
|
|