|
 |
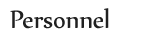 |
|
Thi My Tuyen Nguyen |
The Vi Nguyen |
Van Dat Tuong |
|
|
|
 |
 |
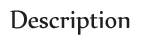 |
|
New MAC Technologies |
Simultaneous media access by multiple devices in any
wireless network can lead to collisions which affects throughput, network efficiency, and other network quality indicators. The management of communication resources using
the medium access control (MAC) protocol is aimed at either avoiding collisions
or resolving them.
|
The core function MAC sub-layer of the Data Link layer
is the management of the transmission medium for multiple devices by employing multiple access techniques to
ensure that the constrained transmission resources are effectively utilised for a successful transmission. The
multiple access techniques are based on rules that indicate
how the transmission resources are allocated and controlled.
These rules are stipulated by the MAC protocol also called
the MAC scheduling protocol.
|
The MAC protocol coordinates multiple nodes sharing wireless transmission medium.
Channel allocation is a typical way to share medium. In particular, wireless channel resource is partitioned into a certain dimension such as time, frequency, and code spreading.
Accordingly, we have time division multiple access (TDMA), frequency division multiple access (FDMA), and code division multiple access (CDMA).
Another important family of MAC protocols are contention-based random access, such as ALOHA, and carrier sensing multiple access with collision avoidance(CSMA/CA).
|
New Multiplexing Technologies |
The MAC protocol relies on the Physical (PHY) layer's
multiplexing mechanism where multiple signals from different devices are multiplexed onto a constrained spectrum
at the same time or different periods. While the PHY layer
multiplexing deals with the combining of signals onto the
transmission resources, the MAC logically deals with the
devices itself using the defined media access rules. Therefore,
the MAC protocol abstracts the access network based on
all the connected devices and all the available transmission
resources to provide the media access rules
|
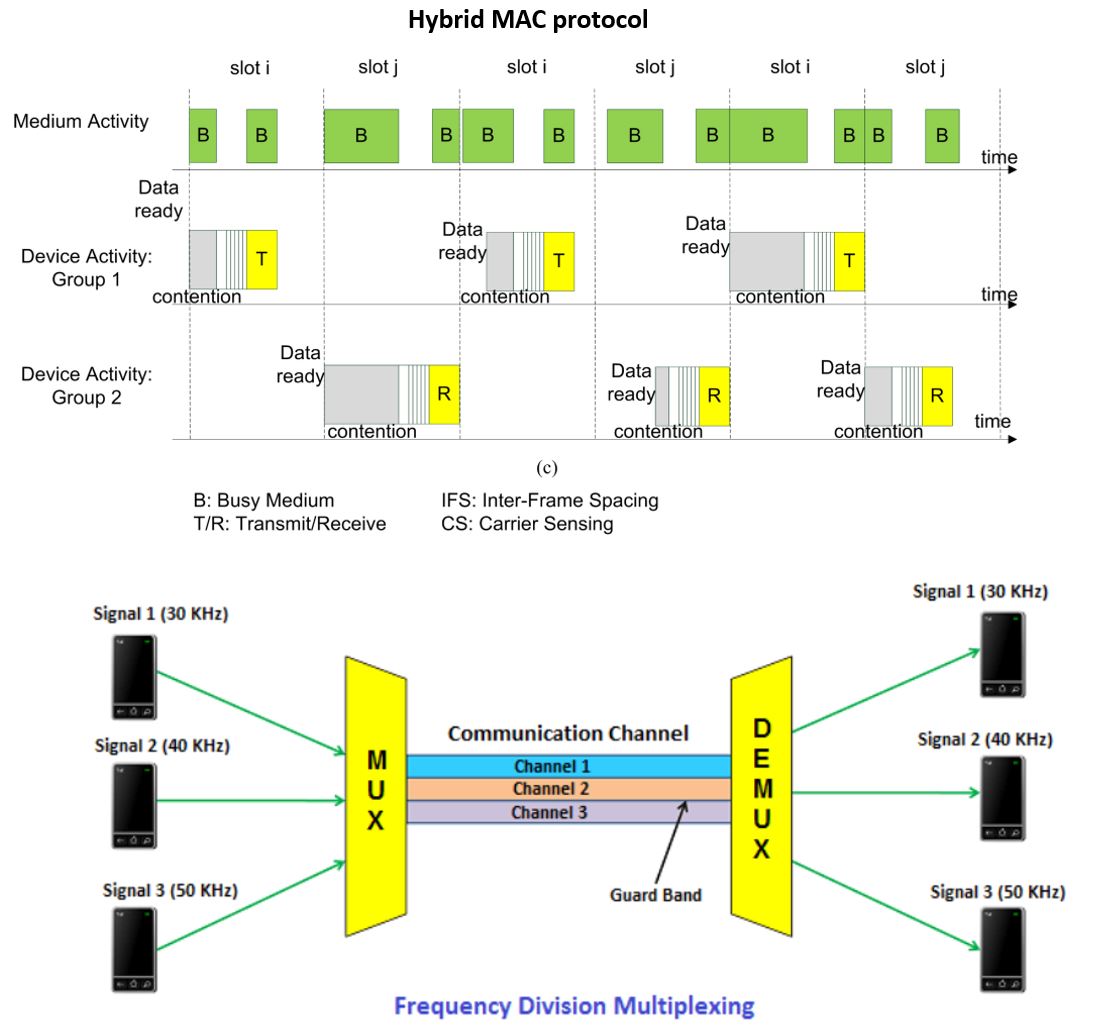 |
Research Topics |
(1) Multiple Access Methods |
(2) Machine Learning-based MAC technologies |
(3) MAC Design for Avoiding Collisions in Large-scale (LS) IoT |
(4) Terahertz MAC Protocols for Nano Communication Networks |
(5) Broader Impact |
|
(1) Multiple Access Methods |
Nonorthogonal multiple
access (NOMA) is regarded as one of the key enabling
technologies to fulfil the requirements of 5G. NOMA can be invoked as an add-on technique for
any existing OMA techniques, such as TDMA/FDMA/
CDMA/OFDMA, due to the fact that it exploits a new
dimension, namely the power domain. Given the
mature status of superimposed coding (SC) and successive interference cancelation (SIC) techniques both in theory
and practice, NOMA may be amalgamated with the
existing MA techniques. Although NOMA has been recognized as a promising candidate for 5G and beyond, there are several research topics worth further investigating.
Specifically, the first challenge is error propagation in SIC. SIC is the key technique of user detection in NOMA systems. Nevertheless, a main drawback of implementing SIC
is the interuser error propagation issue, which propagates
from one user to another, because a decision error results in
subtracting the wrong remodulated signal from the composite multiuser signal, hence resulting in residual interference.
Another interesting topic is how to maintain the sustainability of NOMA with radio frequency (RF) wireless power transfer to improve the energy efficiency of the system.
|
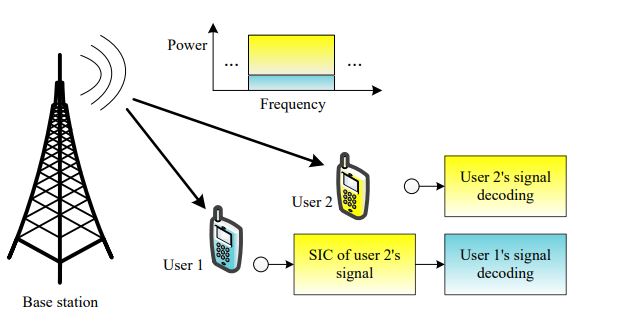 |
|
(2) Machine Learning-based MAC technologies |
To increase the quality of experience (QoE) felt
by end-users, not only network-side but also UE-side
intelligence must be supported in cellular systems. Support
for UE-side intelligence can improve performance at the
medium access control (MAC) layer. Sixth generation (6G) networks aims beyond
"Connected things" to "Connected intelligence" in a more
diverse and dynamic network environment and demands higher
specifications and intelligent communication technologies. Moreover, 6G networks are expected to enable key services, such as extremely reliable and low-latency communications (ERLLC),
ultra-massive machine-type communications (umMTC), long-distance and High Mobility Communications (LDHMC), and Extremely Low-Power Communications (ELPC).
In MAC layer in 6G environment, with the dynamic change and diverse service requirements, optimal resource allocation and random access can be hard to archive.
Machine learning methods are promising for solving these problems, which inherently adapt to dynamic network
environments and provide solutions in real time.
|
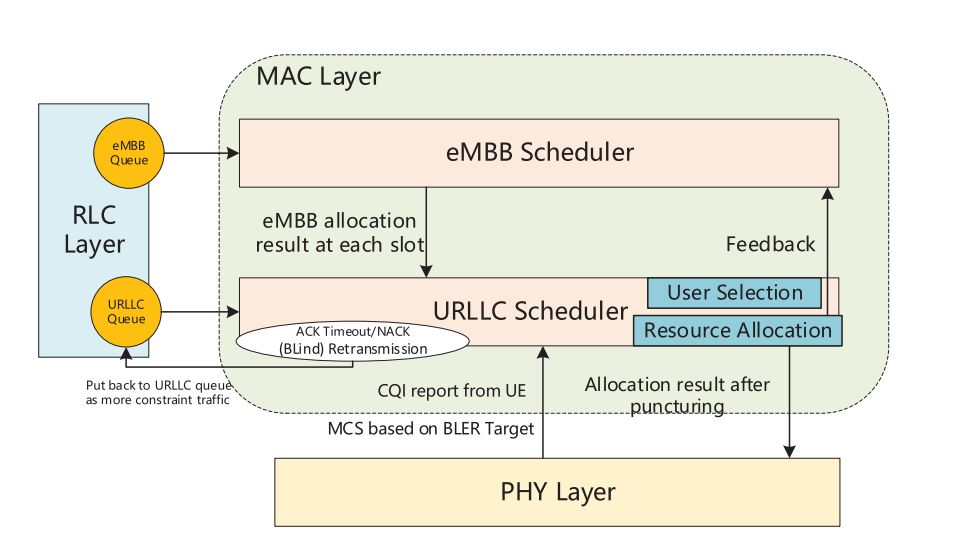 |
|
(3) MAC Design for Avoiding Collisions in Large-scale (LS) IoT |
MAC scalability is the ability of the MAC protocol to efficiently accommodate an increasing number of frame transmissions, reception, and resource allocation. A scalable MAC
ensures seamless multiple media access scheduling for an
extremely large and dynamically growing number of machine-to-machine (M2M)
devices while effectively maintaining QoS requirements of
the large-scale (LS)-IoT network. Scalability of the MAC protocol in LS-IoT networks is
significantly affected by the high collision probability in
contention-based MAC schemes. The collision probability is
established based on the likelihood that there will be at least
one or more devices that may randomly select or compute
the same back-off schedule following the detection of a free medium for a transmission attempt [44]. This likelihood certainly increases for LS-IoT networks because many devices
lead to a high likelihood of transmission or reception of
frames.
|
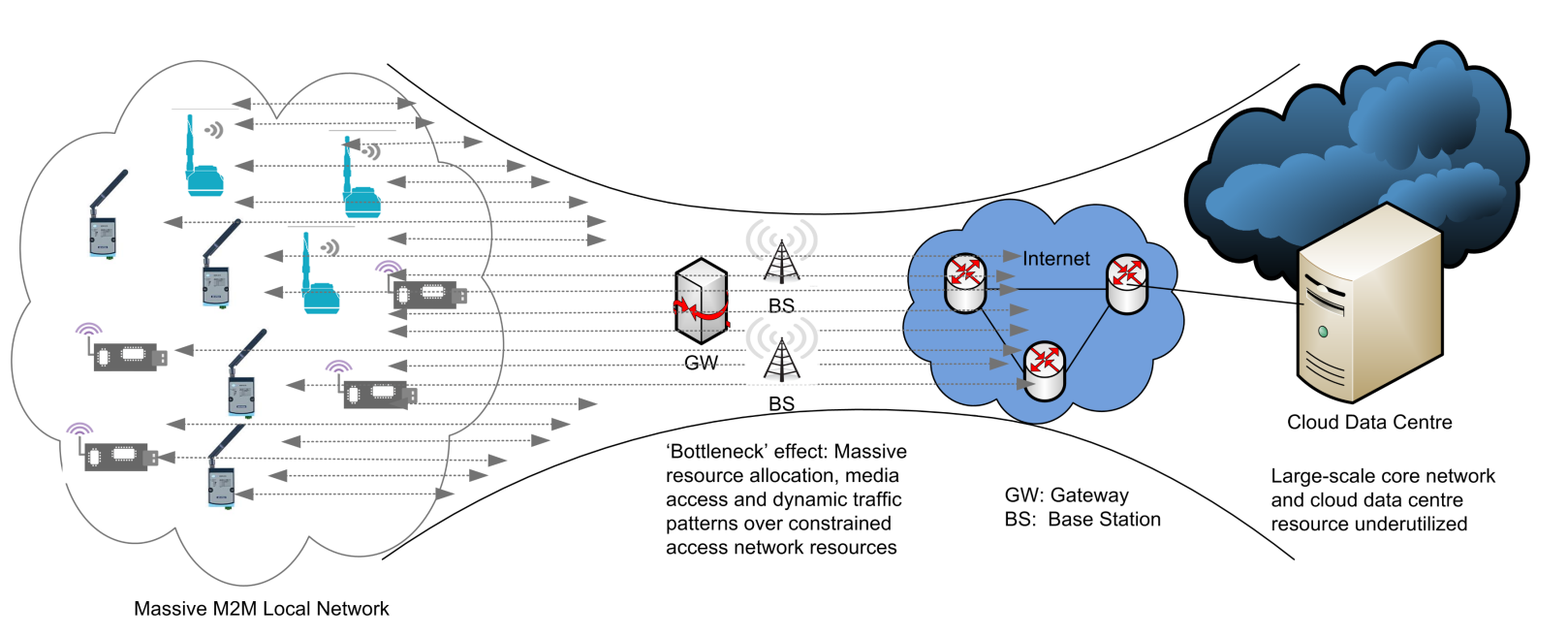 |
|
(4) Terahertz MAC Protocols for Nano Communication Networks |
The nanonetworks include several
nanodevices that work together to perform simple tasks. Due
to limited energy capacity, centralized topology is used in
different applications including In-body networks, air quality
monitoring, and industrial applications. In these applications,
a nano-controller that is capable of performing heavy computation, scheduling and transmission tasks is used. Initially,
nanodevices send their information to the controller, and controller can then process and schedule transmission and sends
information to external networks via a gateway device.
|
In hierarchical architecture, the
network is mainly partitioned in a set of clusters where each
cluster is locally coordinated by a nano controller. The nano
controller is a device that has more processing capabilities of
complex tasks and has high energy availability. Since nanosensor nodes are not capable of processing and handling complex
tasks, these tasks are pushed towards the nano-controllers
which then coordinate their tasks in an efficient manner. The
MAC layer for nano-controller includes more functionalities
such as link establishment and resource allocation.
|
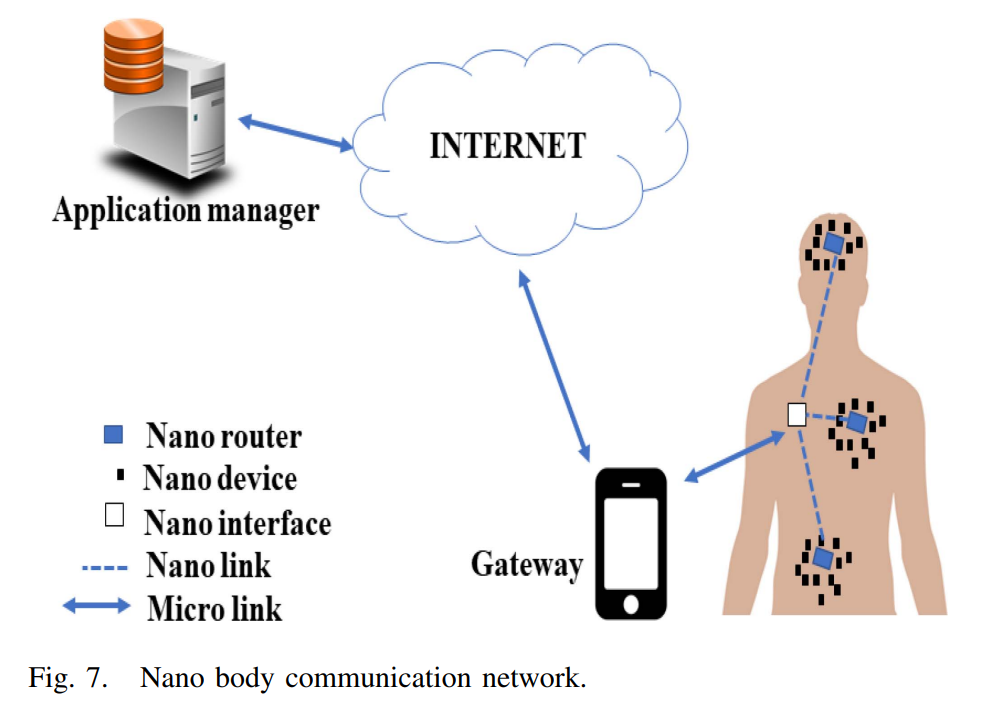 |
|
(5) Broader Impact |
Emerging 5G/6G networks are expected to support various applications and services such as Ultra-reliable low-latency communication (URLLC), enhanced mobile broadband (eMBB).
The new MAC and multiplexing techniques targeting on resource allocation, scheduling control are required to support the stringent requirements of these services.
This project is expected to make significant contributions on discovering novel MAC protocol designs and multiplexing techniques, as well as their applications to improve the network performance.
|
|
|
|
 |
|
 |
 |
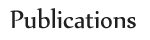 |
|
 |
T. M. T. Nguyen, T.-V. Nguyen, W. Noh, and S. Cho,
"Energy-Efficient and Low-Complexity Transmission Control with SWIPT-NOMA for Green Cellular Networks,"
to appear in IEEE Transactions on Wireless Communications, 2023.
[PDF]
[IEEE Xplore]
|
 |
T. M. T. Nguyen, T. V. Nguyen, D. T. Hua, N. P. Tran, and S. Cho,
"A Survey on Intelligent Reflecting Surface-aided Non-Orthogonal Multiple Access Networks,"
in Proc. of ICTC
, Jeju, Korea, October, 2022.
[PDF] [IEEE Xplore]
|
 |
V. D. Tuong, W. Noh, and S. Cho "
"Delay Minimization for NOMA-enabled Mobile Edge Computing in Industrial Internet of Things,"
IEEE Transactions on Industrial Informatics, vol. 18, no. 10, pp. 7321-7331, Oct. 2022.
[PDF]
[IEEE Xplore]
|
 |
V. D. Tuong, T. P. Truong, T.-V. Nguyen, W. Noh, and S. Cho,
"Partial Computation Offloading in NOMA-Assisted Mobile Edge Computing Systems Using Deep Reinforcement Learning,"
IEEE Internet of Things Journal , vol. 8, no. 17, pp. 13196-13208, Sept. 2021
[PDF]
[IEEE Xplore]
|
|
|
|
 |
|
|
|
|
|